Application and relative importance of spatial analysis for replicated multienvironment yield trials in sugarcane (Saccharum Spp.)
Keywords:
Anisotropic autoregressive, Akaike information criterion, Isotropic autoregressive, Heteroscedastic, Homoscedastic, Crop trials, Relative efficiencyAbstract
Data obtained from multi-environment yield trials of sugarcane are usually analyzed using traditional models that detect only those variations existed between plots or test genotypes. However, blocking does not control spatial heterogeneity existed within and between trials. This investigation was conducted to evaluate the application and relative importance of spatial analysis for sugarcane multi-environment trials conducted under different designs. Yield data obtained from 20 yield trials were analyzed using classical linear and autoregressive models on the basis of mixed model. Results of the within trial analysis indicated both Akaike information criterion (AIC) and relative efficiency selected the classical linear model as best model in most of the cases. The anisotropic autoregressive model was the best model in modeling cane and sugar yields data of plant cane crop trials which received poor management practices and should be applied under these circumstances. When we analyze the combined multi-environment trials data, the heteroscedastic anisotropic autoregressive model showed better adjustments for cane and sugar yield data modeled across locations, while heteroscedastic linear model was the best model for cane and sugar yields data modeled over crop years. Thus, the heteroscedastic isotropic autoregressive model is recommended to be applied in sugarcane METs data analysis. Moreover, our investigation insures the applicability of the spatial analysis for data obtained from yield trials conducted under simple lattice design.References
Berg, V., 1972. Control of the cane sugar manufacture. Manual for sugar chemist course of H.V.A. Wonji-Shoa Sugar Factory, Ethiopia.
Casanoves, F., Macchiavelli, R., Balzarini, M., 2005. Error variation in multi-environment peanut trials: within-trial spatial correlation and between-trial heterogeneity. Tropical Agricultural Research and Higher Education. Crop Sci., 45, 1927-1933.
Cullis, B.R., Gleeson, A.C., 1991. Spatial analysis of field experiments-an extension to two dimensions. Biometrics, 47, 1449-1460.
Edmé, S.J., Tai, P.Y.P., Miller, J.G., 2007. Relative efficiency of spatial analysis for non-replicated early-stage sugarcane field experiments. J. Am. Soc. Sugar Cane Technol., 27, 89-104.
Federer, W.T., Wolfinger, R.D., 1998. SAS code for recovering inter-effect information in experiments with incomplete block and lattice rectangle designs. Agron. J., 90, 545-551.
Gilmour, A.R., Cullis, B.R., Verbyla, A.P., 1997. Accounting for natural and extraneous variation in the analysis of field experiments. J. Agr. Biol. Environ. Stat., 2, 269-273.
Hu, X., Spilke, J., 2009. Comparison of various spatial models for the analysis of cultivar trials. New Zealand J. Agr. Res., 52, 277-287.
Kang, M.S., Balzarini, M.G., Guerra, J.L.L., 2004. Genotype-by-environment interaction. In genetic analysis of complex traits using SAS, edited by A.M., Saxton. Cary, NC: SAS Institute Inc. 69-96.
Kenward, M.G., Roger, J.H., 1997. Small sample inference for fixed effects from restricted maximum likelihood. Biometrics, 53, 983-997.
Magnussen, S., 1990. Application and comparison of spatial models in analyzing tree genetics field trials. Can. J. Forest Res., 20, 536-546.
Mebrahtom, F., 2O17. Genotype x environment interaction, in vitro screening for salinity and drought tolerance in sugarcane (Saccharum officinarum L.). PhD Dissertation in Plant Breeding. School of Plant Sciences, Haramaya University, Ethiopia.
Mebrahtom, F., Firew, M., Eyasu, A., 2018. Genotype x environment interaction and genotype evaluations for yield, yield components and qualities in sugarcane (Saccharum Spp.) Ethiopia. Sci. J. Crop Sci., 7(1), 249-263.
Ostengo, S., Cuenya, I., Balzarini, M., García, M.B., Chavanne, E.R., 2014. TUC 95-10 productive performance analysis by a multi-environment and multi-age mixed model. Rev. Ind. Agr. Tucumán, 91, 25-29.
Ostengo, S., Cunya, M.I., Balzarini, M., 2015. Modeling spatial correlation structure in sugarcane (Saccharum spp.) multi-environment trials. J. Crop Improv., 29, 53-64.
Paget, M.F., Alspach, P.A., Anderson, J.A.D., 2015. Trial heterogeneity and variance models in the genetic evaluation of potato tuber yield. Plant Breed., 1-8.
Schabenberger, O., Pierce, F., 2002. Contemporary statistical models for the plant and soil sciences. Boca Raton, FL: CRC Press.
Singh, M., Chaubey, Y.B., Sarker, A., Sen, D., 2010. Modeling unstructured hetrogeneity along with spatial correlated errors in field trials. J. Indian Soc. Agr. Stat., 64(2), 313-321.
Smith, A., Stringer, J., Wei, X., Cullis, B., 2007. Varietal selection for perennial crops where data relate to multiple harvests from a series of field trials. Euphytica, 157, 253-266.
Smith, A.B., Cullis, B.R., Thompson, R., 2001. Analyzing variety by environment data using multiplicative mixed models and adjustments for spatial field trend. Biometrics, 57, 1138-1147.
Statistical Analysis System Institute, 2009. SAS Users Guide; Version 9.2. SAS Ins. Inc. Cary North Carolina, USA.
Tahir, M., Khalil, H.H., McCord, P.H., Barry, G., 2014. Sugarcane genotype performance in three environments (Based on crop cycle) at Mardan, Pakistan. Am. J. Exp. Agr., 4, 362-375.
Vincenzo, M., 2003. Optimization of experimental design and analysis for sugarcane variety trials. PhD Thesis. The University of Queensland, School of Land, Crop and Food Sciences.
Wolfinger, R.D., Tobias, R.D., Sall, J., 1994. Computing gaussian likelihoods and their derivatives for general linear mixed models. SIAM J. Sci. Comp., 15, 1294-1310.
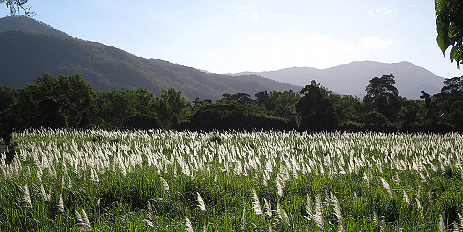
Downloads
Published
How to Cite
Issue
Section
License
Copyright (c) 2020 Mebrahtom Ftwi, Feyissa Taddesse, Astaweskegn Alemu, Melaku Tesfa

This work is licensed under a Creative Commons Attribution-NonCommercial-NoDerivatives 4.0 International License.