Artificial neural networks in foodstuffs: a critical review
Keywords:
Artificial neural networks, Soft computing, Artificial intelligence, Foodstuffs, ReviewAbstract
This paper provides a critical review of literature concerning the artificial neural networks (ANN) in foodstuffs. The main aim is to provide background information, motivation for applications and an exposition to the methodologies employed in the development of ANN techniques in foodstuffs. This review includes that all the latest works on the application of ANN to foodstuffs which have been reported excellently with positive and encouraging results. This review paper highlights the methodologies and algorithms employed for ANN models suitable for various foodstuffs, viz., avocados, tomatoes, cherries, grape, mosambi juice, apple juice, chicken nuggets, pistachio nuts, potato chips, kalakand, cakes, processed cheese, butter, milk and other foodstuffs. This review paper would be very beneficial for those working in food industry, academicians, students, researchers, scientists, factories manufacturing the food products and regulatory authorities, as it provides comprehensive latest information.
References
Amin, M., Navaz, H.K., Kehtarnavaz, N., Dabiri, D., 2009. A systematic approach for solving large-scale problems by
neural network: Open refrigerated display cases and droplet evaporation problems. Food Biopro. Tech. doi:
1007/s11947-008-0167-6.
Benedetti, S., Mannino, S., Sabatini, A.G., Marcazzan, G.L., 2004. Electronic nose and neural network use for the
classification of honey. Apidologie, 35, 1–6.
Campbell, M. R. , Mannis, S. R. , Port, H. A. , Zimmerman, A. M., Glover, D. V., 1999. Prediction of starch amylose
content versus total grain amylose content in corn by near-infrared transmittance spectroscopy. Cereal
Chem. 76(4), 552-557.
Efstathios, Z.P., Fady, R.M., Argyria, A.A., Conrad, M.B., George-John, E.N., 2011. A comparison of artificial neural
networks and partial least squares modelling for the rapid detection of the microbial spoilage of beef fillets
based on Fourier transform infrared spectral fingerprints. Food Micro. 28 (4), 782–790.
García-Gimeno, R.M. , Hervás-Martínez, C., Rodríguez-Pérez, R., Zurera-Cosano, G., 2005. Modelling the growth of
leuconostoc mesenteroides by artificial neural networks. Int. J. of Food Microbio. 105(3), 317–332.
Gori, A., Chiara, C., Selenia, M., Nocetti, M., Fabbri, A., Caboni, M.F., Losi, G., 2011. Prediction of seasonal variation
of butters by computing the fatty acids composition with artificial neural networks. Europ. J. of Lipid Sci. and
Tech. 113(11), 1412–1419.
Goyal, Sumit, Goyal, G.K., 2011a. Advanced computing research on cascade single and double hidden layers for
detecting shelf life of kalakand: an artificial neural network approach. Int. J. of Comp. Sci. and Emerg. Tech.,
(5), 292-295.
Goyal, Sumit, Goyal, G.K., 2011b. Brain based artificial neural network scientific computing models for shelf life
prediction of cakes. Can. J. on A.I., Mach. Learn. Patt. Recogn. 2(6), 73-77.
Goyal, Sumit, Goyal, G.K., 2011c. Simulated neural network intelligent computing models for predicting shelf life of
soft cakes. Global J. Comp. Sci. Tech., 11(14), version 1.0, 29-33.
Goyal, Sumit, Goyal, G.K., 2011d. Development of intelligent computing expert system models for shelf life
prediction of soft mouth melting milk cakes. Int. J. Comp. App. 25(9), 41-44.
Goyal, Sumit, Goyal, G.K., 2011e. Performance of generalized regression, radial basis (fewer neurons), and linear
layer (design) computational ANN techniques for shelf life prediction of processed cheese. Int. J. A.I.
Knowledge Discovery. 1(4), 12-15.
Goyal, Sumit, Goyal, G.K., 2011f. Cascade and feedforward backpropagation artificial neural networks models for
prediction of sensory quality of instant coffee flavoured sterilized drink. Can. J. on A.I., Mach. Learn. Patt.
Recogn. 2(6), 78-82.
Goyal, Sumit, Goyal, G.K., 2011g. Development of neuron based artificial intelligent scientific computer
engineering models for estimating shelf life of instant coffee sterilized drink. Int. J. Comput. Intel. Info. Sec.
(7), 4-12.
Goyal, Sumit, Goyal, G.K., 2011h. Computerized models for shelf life prediction of post-harvest coffee sterilized
milk drink. Lib. Agri. Res. Cen. J. Int. 2 (6), 274-278.
Goyal, Sumit, Goyal, G.K., 2012a. Shelf life determination of kalakand using soft computing technique. Adv. in
Comput. Mathem. and its App. 1(3), 131-135.
Goyal, Sumit, Goyal, G.K., 2012b. Elman backpropagation single hidden layer models for estimating shelf life of
kalakand. Adv. in Info. Tech. and Manag. 1(3), 127-131.
Goyal, Sumit, Goyal, G.K., 2012c. Central nervous system based computing models for shelf life prediction of soft
mouth melting milk cakes. Int. J. Info. Tech. Comp. Sci. 4(4), 33-39.
Goyal, Sumit, Goyal, G.K., 2012d .Linear layer and generalized regression computational intelligence models for
predicting shelf life of processed cheese. Russian J. Agri. Socio-Eco. Sci. 3(3), 28-32.
Goyal, Sumit, Goyal, G.K., 2012e. Shelf life prediction of processed cheese by cascade backpropagation ANN
computing models. Int. J. Electro. Comput. Engg. Edu. 3(1), 23-26.
Goyal, Sumit, Goyal, G.K., 2012f. Supervised machine learning feedforward backpropagation models for predicting
shelf life of processed cheese. J. of Engg. 1(2), 25-28.
Goyal, Sumit, Goyal, G.K., 2012g. Heuristic machine learning feedforward algorithm for predicting shelf life of
processed cheese. Int. J. Basic App. Sci. 1(4), 458-467.
Goyal, Sumit, Goyal, G.K., 2012h. Smart artificial intelligence computerized models for shelf life prediction of
processed cheese. Int. J. Engg. Tech. 1(3), 281-289.
Goyal, Sumit, Goyal, G.K., 2012i. Time – delay simulated artificial neural network models for predicting shelf life of
processed cheese. Int. J. Intel. Sys. App. 4(5), 30-37.
Goyal, Sumit, Goyal, G.K., 2012j. Radial basis (exact fit) and linear layer (design) computerized ANN models for
predicting shelf life of processed cheese. Comp. Sci. J. 2(1), 11-18.
Goyal, Sumit, Goyal, G.K., 2012k. Radial basis (exact fit) artificial neural network technique for estimating shelf life
of burfi. Adv. in Comp. Sci. and its App. 1(2), 93-96.
Goyal, Sumit, Goyal, G.K., 2012l. Soft computing single hidden layer models for shelf life prediction of burfi.
Russian. J. Agri. and Socio-Eco. Sci. 5(5), 28-32.
Goyal, Sumit, Goyal, G.K., 2012m. Time – delay single layer artificial neural network models for estimating shelf life
of burfi. Int. J. Res. Studies in Comp. 1(2), 11-18.
Guyer, D., Yang, X., 2000. Use of genetic artificial neural networks and spectral imaging for defect detection on
cherries. Comp. Electro. Agri. 29(3), 179–194.
Haykin, S.,1994. Neural network: A comprehensive foundation. Canada: Prentice Hall, 22–24.
Lek S., Delacoste M., Baran P., Dimopoulos I., Lauga J., Aulagnier, S., 1996. Application of neural networks to
modeling nonlinear relationships in ecology. Ecol. Model. 90, 39-52.
Maftoonazad, N., Karimi, Y., Ramaswamy, H.S., Prasher, S.O., 2011. Artificial neural network modeling of
hyperspectral radiometric data for quality changes associated with avocados during storage. J. Food Pro.
Preser. 35 (4), 432–446.
Mateo, F., Gadea, R., Medina, A´., Mateo, R., Jime´nez, M., 2009. Predictive assessment of ochratoxin A
accumulation in grape juice based-medium by Aspergillus carbonarius using neural networks. J. App. Micro.
, 915–927.
Movagharnejad, K., Nikzad, M., 2007. Modeling of tomato drying using artificial neural network. Comp. and
Electro. Agri. 59, 78–85.
Omid, M. , Akram, A., Golmohammadi, A., 2011. Modeling thermal conductivity of Iranian flat bread using artificial
neural networks. Int. J. of Food Prop. 14(4), 708-720.
Omid, M., Baharlooei, A., Ahmadi, H., 2009. Modeling drying kinetics of pistachio nuts with multilayer feedforward neural network. Drying Tech.: An Int. J. 27(10), 1069-1077.
Park,S.J., Hwang, C.S., Vlek, P.L.G., 2005. Comparison of adaptive techniques to predict crop yield response under
varying soil and land. Agric. Syst. 85, 59-81.
Qiao, J. , Wang, N. , Ngadi, M.O., Kazemi, S., 2007. Predicting mechanical properties of fried chicken nuggets using
image processing and neural network techniques. J. Food Engg., 79(3), 1065–1070.
Raharitsifa, N., Ratti, C., 2010. Foam-mat freeze-drying of apple juice part 1: Experimental data and ANN
simulations. J. Food Process Engg. 33, 268–283.
Rai, P., Majumdar, G.C., DasGupta, S., De, S., 2005. Modeling the performance of batch ultrafiltration of synthetic
fruit juice and mosambi juice using artificial neural network. J. Food Engg. 71, 273–281.
Sanzogni, L., Kerr, D., 2001. Milk production estimates using feed forward artificial neural networks. Comp. and
Electro. Agri. 32(1), 21–30.
Serpen, A., Gökmen, V., 2007. Modeling of acrylamide formation and browning ratio in potato chips by artificial
neural network. Mol. Nut. Food Res. 51(4), 383–389.
Vinod, K. J., Vikrant, C., 2002. Neural networks approach to modeling food processing operations. In J. M.
Irudayaraj (Ed.), Food process. Oper. Model.: Des. and anal. New York: Marcel Dekker, 1–3.
Yang, C.C., Prasher, S.O., Landry, J.A., Ramaswamy, H.S, Ditommaso, V., 2000. Application of artificial neural
networks in image recognition and classification of crop and weeds. Can. Agri. Engg., 42 (3), 147-152.
Zhang, Q., Yang, S.X., Mittal, G.S., Yi, S. (2002). Prediction of performance indices and optimal parameters of rough
rice drying using neural networks. Biosys. Eng. 83, 281-290.
Zurera-Cosano, G., García-Gimeno, R.M., Rodríguez-Pérez, M.R., Hervás-Martínez, C., 2005. Validating an artificial
neural network model of leuconostoc mesenteroides in vacuum packaged sliced cooked meat products for
shelf-life estimation. Europ. Food Res. Tech. 221(5), 717-724.
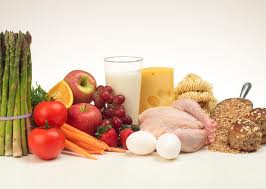
Published
How to Cite
Issue
Section
Copyright (c) 2012 S. Goyal, G. K. Goyal

This work is licensed under a Creative Commons Attribution-NonCommercial-NoDerivatives 4.0 International License.